Course Description
Data Science Certification from SGIT, Steinbeis University, Germany:
Accelerate your career with Data Science certification from SGIT, Steinbeis University Germany , one of the leading universities in Germany. This course is a perfect blend of theory, case studies and capstone projects. The course curriculum has been designed by Steinbeis University and considered to be the best in the industry. Get noticed by recruiters across the globe with the international certification. Post certification one will gain the alumnus status in Steinbeis University.
What is the certification process?
Post completion of the training, one should take an online examination facilitated by the university and should attain 60% or more to complete the course and gain the certification. Subsequently participants can check their alumnus status on SGIT , Steinbeis Global Institute Tübingen.
Advanced Certification Program in Data Science and AI for Digital Transformation from IITM Pravartak:
ExcelR, in association with IIT Madras, brings to you an add-on certification for your Data Science Course.
This certification program provides you with:
- 15+ Hours of Interactive Live-Virtual Sessions by professors of IIT Madras.
- Optional 2-day Campus Immersion in the beautiful, state-of-the-art IIT Madras.
- A prestigious IIT Madras Pravartak Certificate.
What is the certification process?
During the period of your course, interactive live-virtual sessions will be conducted by professors of IIT Madras. An optional campus immersion will also be planned, whereby a slot will be created, and you will travel to Chennai for a two-day experience at the IIT Madras campus. Post training, you will take a short quiz on the topics discussed in the session, which will unlock your Advanced Certification Program in Data Science and AI for Digital Transformation from IITM Pravartak.
Data Science Course Training
ExcelR offers Data Science course, the most comprehensive Data Science course in the market, covering the complete Data Science lifecycle concepts from Data Collection, Data Extraction, Data Cleansing, Data Exploration, Data Transformation, Feature Engineering, Data Integration, Data Mining, building Prediction models, Data Visualization and deploying the solution to the customer. Skills and tools ranging from Statistical Analysis, Text Mining, Regression Modelling, Hypothesis Testing, Predictive Analytics, Machine Learning, Deep Learning, Neural Networks, Natural Language Processing, Predictive Modelling, R Studio, Tableau, Spark, Hadoop, programming languages like R programming, Python are covered extensively as part of this Data Science training. ExcelR is considered as the best Data Science training institute which offers services from training to placement as part of the Data Science training program with over 400+ participants placed in various multinational companies including E&Y, Panasonic, Accenture, VMWare, Infosys, etc. ExcelR imparts the best Data Science training and considered to be the best in the industry.
Why Should You Choose ExcelR For Data Science Training?
If you are serious about a career pertaining to Data science, then you are at the right place. ExcelR is considered to be one of the best Data Science training institutes. We have built careers of thousands of Data Science professionals in various MNCs in India and abroad. “Training to Job Placement” – is our niche. We do the necessary hand-holding until you are placed. Our expert trainers will help you with upskilling the concepts, to complete the assignments and live projects.
ExcelR has a dedicated placement cell and has partnered with 150+ corporates which will facilitate the interviews and help the participants in getting placed. ExcelR is the training delivery partner in the space of Data Science for 5 universities and 40+ premier educational institutions like IIM, BITS Pilani, Woxen School of Business, University of Malaysia, etc. Faculty is our strength. All of our trainers are working as Data Scientists with over 15+ years of professional experience. Majority of our trainers are alumni of IIT, ISB and IIM and a few of them are PhD professionals. Owing to our faculty, ExcelR’s certification is considered to be the best Data Science certification offered in this space. ExcelR offers a blended learning model where participants can avail themselves classroom, instructor-led online sessions and e-learning (recorded sessions) with a single enrollment. A combination of these three modes of learning will produce a synergistic impact on learning. One can attend an unlimited number of instructor-led online sessions from different trainers for 1 year at no additional cost. No wonder ExcelR is regarded as the best Data Science training institute to master Data Science concepts and crack a job.
What Is Data Science? Who Is Data Scientist?
Data Science is all about mining hidden insights of data pertaining to trends, behaviour, interpretation and inferences to enable informed decisions to support the business. The professionals who perform these activities are said to be a Data Scientist / Science professional. Data Science is the most high-in-demand profession and as per Harvard and the most sort after profession in the world.
Why One Should Take The Data Science Course?
Is Data Science certification being worth pursuing as a career?
The answer is a big YES for myriad reasons. Digitalization across the domains is creating tons of data and the demand for the Data Science professionals who can evaluate and extract meaningful insights is increasing and creating millions of jobs in the space of Data Science. There is a huge void between the demand and supply and thereby creating ample job opportunities and salaries. Data Scientists are considered to be the highest in the job market. Data Scientist career path is long-lasting and rewarding as the data generation is increasing by leaps and bounds and the need for the Data Science professionals will increase perpetually.
- 1.4 Lakh jobs are vacant in Data Science, Artificial Intelligence and Big Data roles according to NASSCOM
- The world will notice a deficit of 2.3 Lakh Data Science professionals by 2021
- The Demand for Data Scientist professionals has increased by 417% in the year 2018, in India, as per the Talent Supply Index
- Data Science is the best job to pursue according to Glassdoor 2018 rankings
- Harvard Business Review stated that ‘Data Scientist is the sexiest job of the 21st century’
You May Question If Data Science Certification Is Worth It?
The answer is yes. Data Science / Analytics creating myriad jobs in all the domains across the globe. Business organizations realised the value of analysing the historical data in order to make informed decisions and improve their business. Digitalization in all the walks of the business is helping them to generate the data and enabling the analysis of the data. This is helping to create myriad data science/analytics job opportunities in this space. The void between the demand and supply for the Data Scientists is huge and hence the salaries pertaining to Data Science are sky high and considered to be the best in the industry. Data Scientist career path is long and lucrative as the generation of online data is perpetual and growing in the future.
Why ExcelR Is The Best Data Science Training Institute?
ExcelR offers the best Data Science certification online training along with classroom and self-paced e-learning certification courses. The complete Data Science course details can be found in our course agenda on this page.
Who Should Do The Data Science Course?
Professionals who can consider Data Science course as a next logical move to enhance in their careers include:
- Professional from any domain who has logical, mathematical and analytical skills
- Professionals working on Business intelligence, Data Warehousing and reporting tools
- Statisticians, Economists, Mathematicians
- Software programmers
- Business analysts
- Six Sigma consultants
- Fresher from any stream with good Analytical and logical skills
Interview Preparation Sessions
Participants who have completed the Data Science course training and the projects will be put under our Placement Incubation Program. As part of this program, participants will undergo a thorough interview preparation process on Data Science. A huge repository of Data Science Interview questions with answers will be provided for the participants to prepare. A dedicated Data Science Subject Matter Expert (SME) will help in resume building, conduct mock interviews and evaluate each participant's knowledge, expertise and provide feedback. Our SMEs will do the necessary handholding on interview preparation process till the time the participant is placed. Guidance is also provided on Linkedin profile building and tricks of the trade to improve the marketability of the resume. - ExcelR Management
Projects
- As more and more people are expressing their views and opinions on various microblogging websites about various products and services. There has been a surge of data generated by the users, these websites have people sharing their thoughts daily.
- Sentiment Analysis with the help of Natural Language Processing technique for identifying the sentiments of a product or service
- Customers are looking for more information before buying a product on E-commerce websites. Amazon introduced a new feature 'question and answer' search field for products.
- The project is to build an information retrieval system from Amazon products data based on NLP techniques. Top 5 relevant answers to be retrieved based on input question
- Reducing the risk of fraudulent loans by carefully evaluating the risk & at the same time increasing profits by rejecting only those loans, which have the potential of defaulting
- The objective of the analysis to predict an item when sold, what is the probability that customer would file for warranty and to understand important factors associated with them
- Predict which flights would be delayed and by how long?
- Flight delays cost the industry an estimated $25 billion every year More than 60 percent of frequent flyers cite delays among the things about air travel that they find most dismaying. And the costs are spread around - an extra $25 in parking here, a missed business meeting there. Carriers, meanwhile, pay an estimated $62 per minute in crew, fuel, maintenance and other costs. It adds up.
Career Progression and Salary Trends
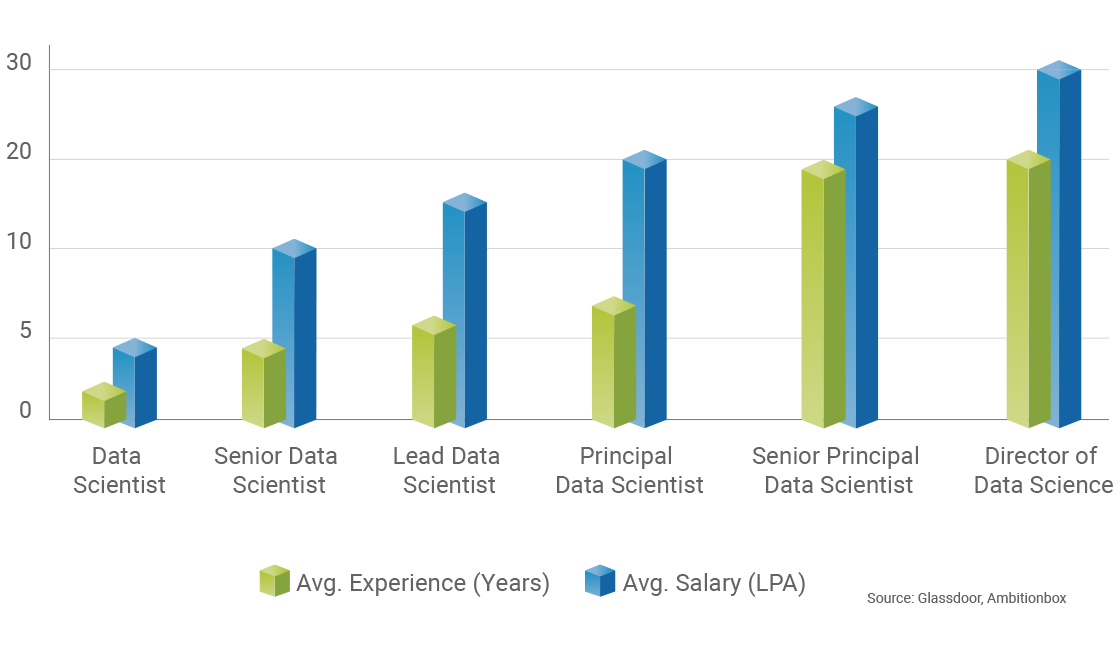
Learning Path
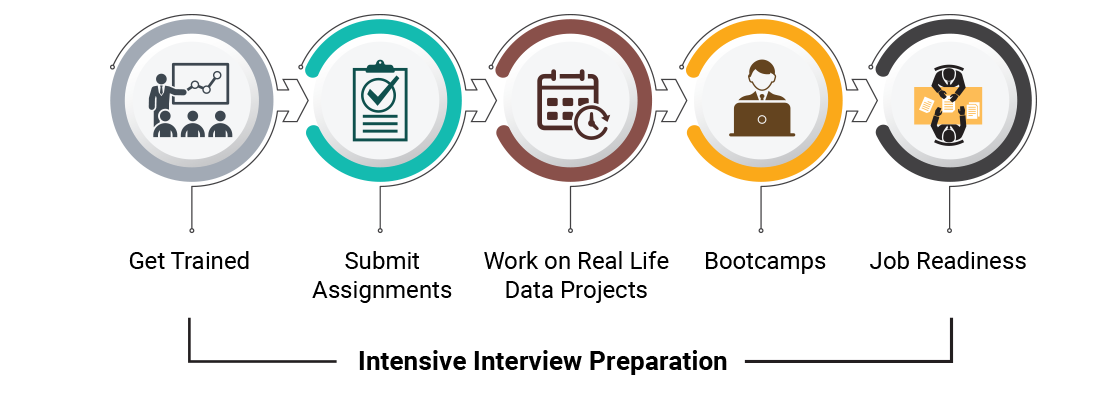
Course Curriculum
Data Science
- Data Types
- Measure Of central tendency
- Measures of Dispersion
- Graphical Techniques
- Skewness & Kurtosis
- Box Plot.
- Random Variable
- Probability
- Probability Distribution
- Normal Distribution
- SND
- Expected Value
- Sampling Funnel
- Sampling Variation
- Central Limit Theorem
- Confidence interval
- Introduction to Hypothesis Testing
- Hypothesis Testing ( 2 proportion test, 2 t sample t test)
- Anova and Chi Square
- Principles of Regression
- Intro to Simple Linear Regression
- Multiple Linear Regression
- Logistic Regression
- Data Cleaning
- Imputation Techniques
- Data analysis and Visualization
- Scatter Diagram
- Correlation Analysis
- Transformations
- Encoding Methods - OHE, Label Encoders,Outlier detection-Isolation Forest and Calculating the Predictive Power Score (PPS)
- Clustering introduction
- Hierarchical clustering
- K Means
- DBSCAN
- PCA
- Association Rules
- Recommender System
- Python Model Deployment
- Regression Tasks / Classification Tasks
- Decision Tree
- KNN
- Support Vector Machines
- Feature Engineering (Tree based methods, RFE,PCA)
- Model Validation Methods (train-test,CV,Shuffle CV, and Accuracy methods)
- Lasso and Ridge Regressions
- ANN
- Optimization Algorithm(Gradient descent)
- Stochastic gradient descent(intro)
- Back Propagation method
- Introduction to CNN
- Bagging and Random Forest
- Boosting
- XGBM
- LGBM
- ntroduction to Text Mining
- VSM
- Intro to word embeddings
- Word clouds and Document Similarity using cosine similarity
- Named Entity Recognition
- Text classification using Naive Bayes
- Emotion Mining
- Introduction to Time Series
- Level
- Trend and Seasonality
- Strategy
- Scatter plot
- Lag plot
- ACF
- Principles of Visualization
- Naive forecasts
- Forecasting Error and it metrics
- Model Based Approaches
- AR Model for errors
- Data driven approaches
- MA
- Exponential Smoothing
- ARIMA
Core Python
- Python Introduction- Programing Cycle of Python,PythonIDE and Jupyter Notebook
- Variables
- DataType
- Github
- HackerRank
- CodeWars and Sanfoundry Account Creation Number
- String
- List
- Tuple
- Dictionary
- Operator-Arithmetic
- Comparison
- Decision Making-Loops
- While Loop
- For Loop and Nested Loop
- Number Type Conversion-int(), long().Float()
- Strings-EscapeChar
- String Special Operator
- String Formatting Operator
- Python List
- Accessing values in list
- Delete list elements
- Indexing, Slicing & Matrices
- Tuples
- Accessing values in Tuples
- Delete Tuples elements
- Indexing
- Slicing & Matrices
- Dictionary
- Accessing Values from Dictionary
- Deleting and Updating Elements in Dict
- Properties of Dist
- Built-In Dist Functions & Methods
- Dict Comprehension
- Function
- Define Function
- Calling Function
- Pass by Reference as Value
- Function Arguments
- Anonymous Functions
- Return Statements
- Scope of Variables
- Local & Global
- Decorators and Recursion
- Import Statements
- Locating Modules
- Current Directory
- Python path
- Dir() Function
- Global and Location Functions & Reload() Functions
- Sys Module and Subprocess Module
- Packages in Python
- Files in Python
- Reading Keyboard Input
- Input Function
- Opening and Closing Files
- Syntax and List of Modes
- Files Object Attribute Open,Close.
- Reading and Writing Files
- File Position Directories Mkdir Method
- Chdir() Method
- Getcwd Method
- Rmdir
- Exception Handling
- List of Exceptions
- TryandException
- OOP Concepts, Class, Objects, Inheritance, Overriding Methods like __init__, Overloading Operators, Data Hiding
- Match Function
- Search Function
- Matching Vs Searching
- Regular Exp Modifiers and Patterns
- Database Connectivity
- Methods
- MySQL
- Oracle
- How to Install MySQL
- DB Connection
Tableau
- What is Tableau ?
- What is Data Visualization ?
- Tableau Products
- Tableau Desktop Variations
- Tableau File Extensions
- Data Types
- Dimensions
- Measures
- Aggregation concept
- Tableau Desktop Installation
- Data Source Overview
- Live Vs Extract
- Bar Chart
- Pi-Chart
- Heat Maps
- Histogram
- Maps
- Scatterplot
- Donut Chart
- Waterfall Chart etc..
- Dual axis
- Blended axis
- Dimension Filter
- Measure Filter
- Data Source Filter
- Extract Filter
- Context Filter
- Quick Filter
- Basic Calculations
- Table Calculations
- Quick Table Calculations
- LOD's
- KPI's
- Joins
- Relationship
- Data Blending
- Union
- Hierarchy
- Group
- Sets
- Parameters
- Reference Lines
- Trend Line
- Forecasting
- Clustering
- Dashboard Objects
- Dashboard Actions
- Tableau Public website
MySQL
- Introduction to Databases
- Introduction to RDBMS
- Different types of RDBMS
- Software Installation(MySQL Workbench)
- Data Definition language
- Data Manipulation Language
- Data Query Language
- Transactional Control Language
- Data Control Language
- SELECT
- LIMIT
- DISTINCT
- WHERE
- AND
- OR
- IN
- NOT IN
- BETWEEN
- EXIST
- ISNULL
- IS NOT NULL
- WILD CARDS
- ORDER BY
- GROUP BY
- HAVING
- COUNT
- SUM
- AVG
- MIN
- MAX
- COUNT
- String Functions
- Date & Time Function
- NOT NULL
- UNIQUE
- CHECK
- DEFAULT
- ENUM
- Primary key
- Foreign Key (Both at column level and table level)
- Inner
- Left
- Right
- Cross
- Self Joins
- Full outer join
- Index
- View
- Sub-query
- Window Functions
- Stored Procedures
- Exception Handling
- Loops
- Cursor
- Triggers
Artificial Intelligence
- Introduction
- DeepLearningImportance[Strength & Limitation]
- SP | MLP Neural Network Overview
- Neural Network Representation Activation Function
- Loss Function Importance of Non-LinearActivation Function
- Gradient Descent for NeuralNetwork
- Train
- Test & Validation Set
- Vanishing &ExplodingGradient
- Dropout Regularization
- OptimizationAlgo
- LearningRate
- Tuning
- Softmax
- CNN
- Deep Convolution Model
- Detection Algorithm
- CNN FaceRecognition
- RNN
- LSTM
- BiDirectionalLSTM
Big Data Tools
- Introduction to BigData, Challenges in Big Data and Workarounds| Introduction to Hadoop and Its Components|HadoopComponents andHands-On|Understand the Map Reduce and ItsDrawbacks
- Introduction to Spark and DataBricks|Spark Components, Spark MLlib Spark &DataBricks andHands-On One ML Model in Spark
- Cloud Computing
- Azure Cloud Platform
- Cloud Applications
- Cloud Services
- Open AI Studio
Basics of R
- Data Structures & Operators in R|Conditional Statement|Decision Making|Loops|Strings|Functions|How to Import Data set in R| Programming Statistical Graphics
ChatGPT
- Introduction to ChatGPT and AI
- Types of AI and ChatGPT architecture
- ChatGPT Functionalities and Applications
- ChatGPT Prompt Engineering
Contact Our Team of Experts